
Writing a Questionnaire for a Conjoint Analysis Study
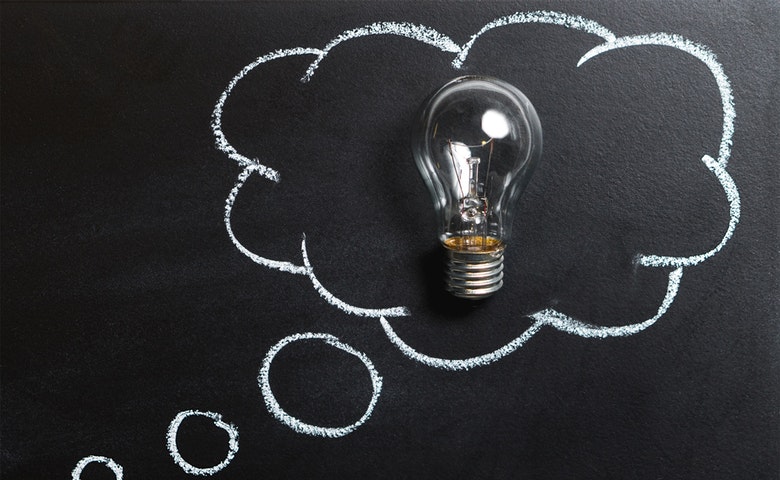
The hard bit of designing a choice-based conjoint analysis (choice modeling) study is creating the experimental design. However, there are a few others parts of a conjoint analysis study which are non-standard and can be tricky. This post gives you ten tips for creating a basic outline of a questionnaire for your choice-based conjoint analysis study.
Screener
The first section of a questionnaire contains the screening questions, which are used to determine if people fulfill the criteria for eligibility to the study (e.g., are users of a particular product), as well as for collating quota information (i.e., ensuring an adequate cross-section of people in key sub-groups). For example, most commercial studies are only open to people aged 18 or more, and have quotas in terms of age, gender, and geography, as well as whatever other screeners are applicable to the study of interest.
Explain how to do the first choice question
It is usually desirable to get people into the choice questions (also known as choice tasks) as soon as possible. This is because these questions are both taxing and boring, so you want people to be answering them when their minds are as fresh as possible.
When the first question is presented, or, immediately prior to this, it is usually useful to:
- Provide relevant background information. For example, if your study is in a technology market and the choice model has attributes containing new technologies, it is usually a good idea to introduce these new technologies and ask questions that test comprehension immediately prior to the choice-based conjoint analysis questions.
- Encourage honesty and attention. For example, “Your answers to this questionnaire will help determine what you can buy, so the more attention you devote to the survey, the more likely you are to be able to buy what you want”.
(Optional) Ask people to explain their first choice
It can be helpful ask people to explain how they made their first choice. Ideally, this question will appear on the same screen immediately after they have made their first choice. This has two goals. One is to reinforce that the respondent’s choices are important. The other is as a way of verifying that your data is good. For example, if you have a study which includes attributes for brand and price, and the person says something like “I chose the Chevrolet because it is a brand that I know will always have good prices”, then you know you have a bit of a problem, as the assumption in a choice model is that people will rely on the attribute descriptions when making their choices.
Explain that the following choice questions vary
After the first question it is usually a good idea to put in a screen saying something like “Now we are going to show you 11 questions, just like the one you completed. On each question the descriptions of the alternatives change, so please read them carefully.” Without such a question, some respondents assume that there is an error and that they have been asked the same question twice!
Count down the number of choice questions
As you ask each choice question it is a good idea to make it clear how many have been done and how many are left to be done (e.g., question 3 of 12). This is because the questions are boring, and you do want to make people feel like it will not go forever. If you are doing a lot of questions, say more than 10, it may be a good idea to give the respondent a break in the middle (e.g., “Nice work. You are half way done!”).
Situational data and cueing
In some markets the situation a person is in has a large impact on their choices. In alcohol markets, what people choose when going to a friend’s house is often different to what they drink at home. In transportation studies, where people live is a big determinant of their options. In such markets it is usually useful to:
- Collect detailed information about the situation, including information regarding the specific attribute levels currently available to them (e.g., in a study of transport choice, collecting data on fuel costs, travel times, etc.).
- Occasion cue the choice questions. That is, rather than asking “which would you choose”, instead saying something like “Now thinking back to what you did last Saturday night, if you had the following options available to you instead, which would you have chosen?” When occasion-cuing it is generally preferable to ask people about only one occasion, as none of the standard choice models are applicable to dealing with situations where people are asked about multiple occasions.
Collect data for validation purposes
There are two types of information that can be useful when checking a choice model. First, it is useful to collect data that you expect to be correlated with the estimated utilities. For example, in a market with luxury goods, you may want to collect income or status consciousness, and check that these are correlated with the estimated utilities. Second, it is usually a good idea to check information about current and historic behavior (e.g., brands previously purchased, last purchased, how often the consumer switches), so that you can verify and calibrate the model See 12 Techniques for Increasing the Accuracy of Forecasts from Choice Experiments for more information.
Profiling questions
There will usually be additional data requirements, such as demographics and attitudinal data.
Start and end-time information
It is usually helpful to collect data on the start and end-time, and, if possible, on the time taken to answer each of the choice questions. This is useful for identifying people that have completed the questionnaire in an impossibly short amount of time, so such people can be excluded from further analyses.
Versions and tasks
Lastly, but most importantly, you need to make sure that the questionnaire is programmed in such a way that you capture data about which question were answered in which order by which people. Without this information, there is no way to analyze the data!
I hope you found this information helpful!