
How to Work Out the Number of Segments For a Market Segmentation
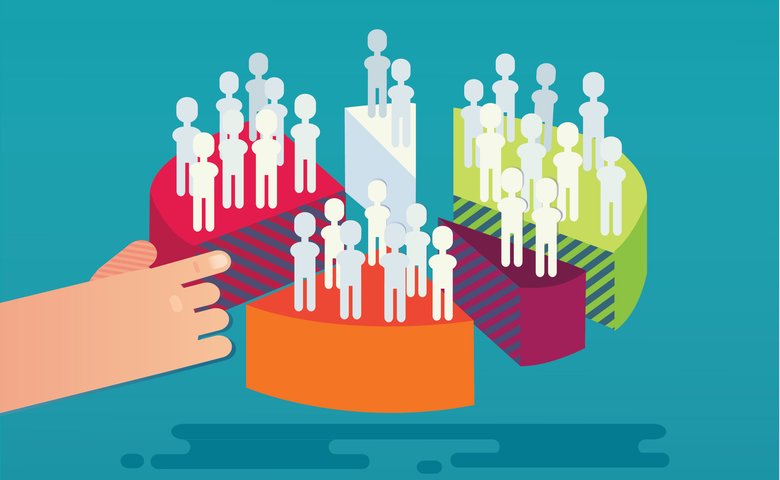
When segmenting a market, a practical challenge is to work out the number of segments. There are eight approaches to choosing the number of segments: strategic usefulness, no small segments, extent of association with other data, cross-validation, penalized fit heuristics, statistical tests, entropy, and replicability.
Strategic usefulness
On choosing the number of segments, the key determinant is typically which number of segments leads to a solution that seems to have the most useful strategic and practical implications. This is usually judged best by the end-users of the segmentation, as this is a managerial rather than a statistical question.
The no small segments and extent of association with other data methods are both closely related to strategic usefulness.
No small segments
The basic idea of this approach is that you choose the highest number of segments, such that none of the segments are small (less than 5% of the sample). This rule has two justifications. One is that solutions with very small segments are unlikely to be statistically reliable. The other is that small segments are unlikely to be strategically usable.
A weakness of this approach is the difficulty of justifying the choice of cutoff value.
Extent of association with other data
This approach involves assessing the extent to which each number of segments solution (i.e., the two-segment solution, the three-segment solution, etc.) are associated with other data. There are two rationales for this approach. One is that the stronger the association with other data, the greater the likelihood that the solution is valid, rather than just reflecting noise. The second is that if a solution is not associated with other data, it will be difficult to use in practice (e.g., it will be difficult to target advertising and distribution if the segmentation is not related to variables that are correlated with advertising usage and shopping behavior).
A practical challenge with this approach is that any truly novel and interesting finding is one that does not relate strongly to existing data.
Cross-validation
This approach involves using only a subset of the data for each subject (or whatever other unit of analysis is used) when fitting a model for a specified number of segments. Subsequently, it involves computing some measure of fit (e.g., log-likelihood) of the fitted model with the observations not used in estimation. This is repeated for different numbers of segments (e.g., from 1 to 10), and the number of classes with the best fit is selected. A variant of this, called K-fold cross validation, instead operates by splitting the sample into K groups and estimating K models for the K-1 groups and judging them based on the fit for the K groups.
The strength of these approaches is that they rely on few assumptions. The weakness is that when there is little data, or only small differences between the number of segments, these approaches are not highly reliable.
Penalized fit heuristics
Provided there are no technical errors, it should always be the case that the more segments you have, the better the segments will fit the data. At some point, however, adding the number of segments will overfit the data. Penalized fit heuristics are metrics that start with a computation of fit, and then penalize this based on the number of clusters.
Dozens and perhaps hundreds of penalized fit heuristics have been developed, such as the Bayesian information criteria (BIC), the gap statistic, and the elbow method (where the penalty factor is passed on the perceptions of the analyst rather than as a cut-and-dried rule).
A practical challenge with all penalized fit heuristics is that they tend to be optimized to work well for a very specific problem but poorly in other contexts. As a result, such heuristics are not in widespread use.
Statistical tests
Statistical tests, such as likelihood ratio tests, can also be used to compare different number of segments, where the difference distribution is bootstrapped.
Practical problems with this approach include that it is highly computationally intensive, that software is not widely available, and that the local optima that inevitably occur mean that the bootstrapped likelihood ratio test will inevitably be highly biased.
Entropy
When latent class analysis is used for segmentation, an output from latent class analysis is an estimate of the probability that each subject (e.g., person) is in each of the segments. This data can be summarized into a single number, called entropy, which takes a value of 1 when all respondents have a probability of 1 of being in one class, and value of 0 when the probabilities of being assigned to a class are constant for all subjects. (Sometimes this is scaled in reverse, where 0 indicates all respondents have a probability of 1 and 1 indicates constant probabilities.)
The main role of entropy is to rule out the number of segments when the entropy is too low (less than 0.8). The evidence in favor of selecting the number of segments based on entropy is weak.
Replicability
Replicability is computed by either randomly sampling with replacement (bootstrap replication) or splitting a sample into two groups. Segmentation is conducted in the replication samples. The number of segments which gets the most consistent results (i.e., consistent between the samples) is considered to be the best. This approach can also be viewed as a form of cross-validation.
Two challenges with this approach are that local optima may be more replicable than global optima (i.e., it may be easier to replicate a poor solution than a better solution), and that replicability declines based on the number of segments, all else being equal.