
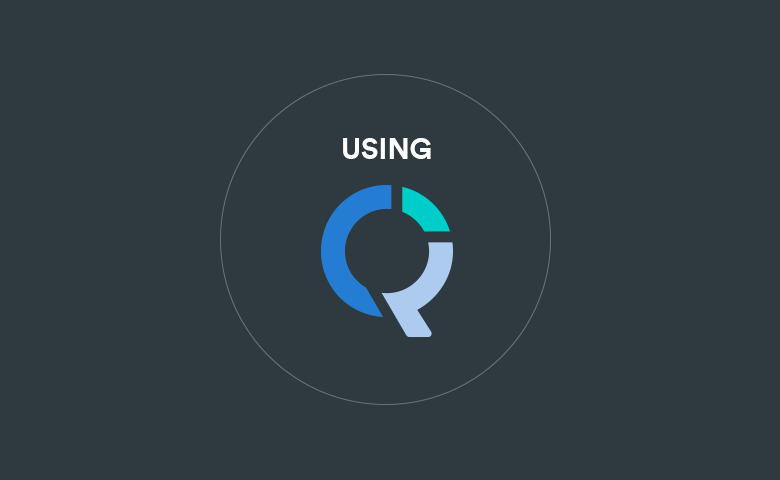
If you can get an SPSS file for your study then Q will usually be able to set up all the data for you. However, if you get stuck with an Excel file you may find yourself having to do a bit more to get started with the data. Why is that? The reason is that your SPSS files contain metadata that tells Q more about how your questions were asked in the survey and hence how Q should set them up.
In an Excel file (.xlsx or .csv) there is no metadata – all Q gets to see are the contents of each column. If you have some control over the contents of the Excel file then you can optimize your experience using that data in Q with a few simple guidelines.
This article covers two main areas:
- How to properly format an Excel-style file for Q
- How to set things up in Q after importing the data
For both phases, it is important that you have a copy of the questionnaire on hand so that you can be confident about changes that you make in Excel or Q.
Formatting Excel files for Q
The basic layout of your Excel file is as follows, and an example of what it should look like is included in the picture below:
- All of your data should be in one worksheet. Q won’t be able to use data in multiple worksheets.
- Each variable from your survey should have its own column (e.g., Age is in one column, Education is in another).
- The first row of the Excel file should have the names for each variable. Make sure these headings begin with letters or words, not numbers or symbols (#!?&), and that make sure these headings describe what is in each column (e.g., Age or Q1. Age rather than just Q1). This will make things a lot easier when the data is in Q.
- Each person’s responses should be contained in a separate row.
- Where possible, ensure that categories are stored as labels rather than numbers (e.g. use Male and Female instead of 1 and 2 in the column for the gender question).
- You should not have any merged cells in the table.
In the next section, I will explain the best way to arrange the contents of your columns for different types of data.
Setup for different data types
While Excel files do not have metadata to tell Q how to set up the data, Q will try to infer the structure of your data based on what is in the columns and column headers. There are some conventions that you can use that will help reduce the amount of setup that you need to do once the file is imported.
Categorical data
When a column contains categories, it is almost always better that the column contains the labels of the categories rather than numbers which indicate the category values. The reason is that if the labels are not present, you will need to enter them later. If you’re stuck with numbers in your file, you can use Excel’s find-and-replace feature to replace values within individual columns or even groups of columns.
Multiple-response data
When you have a multiple response question, the data should be stored in the Excel file with one column for each of the possible options that were shown in the survey. Be sure to avoid storing multiple responses within a single column, as this will make the data very difficult to analyze in Q.
An example of the proper layout for a multiple response question is shown above in the last three columns. The columns headed as Q1a, Q1b, and Q1c represent three options that were shown in a multiple-response question. More tips for column headers for this type of data are considered below.
Missing data and ‘Don’t know’ categories
When a respondent has not given an answer to a question or skipped the question, then they should have an entry of NA in that cell. When working with categories, other kinds of responses which indicate the respondent saw the question but did not give an answer are generally best given a label of Don’t know or whatever phrasing matches your questionnaire.
Good conventions for column headers
Above I mentioned that your headers should always start with a letter rather than a number and that they should be as descriptive as possible. Here I’ll take a deeper look at some common cases where improving the headers will make a big difference.
Ditch multiple header rows
Some online survey providers will export an Excel or CSV file with two or more header rows describing the data. Here is one such example that actually contains three header rows:
The respondent data does not begin until the fourth row in the file. The first row contains short names, the second row contains the full question text, and the third row contains some additional tags.
Before using a file like this in Q you must reduce it to one header. In some cases, you may simply delete the rows that you don’t need. In other cases, you might like to use the Excel CONCATENATE() function to create a new header row. CONCATENATE() is used to paste together two or more pieces of text, and so could be used to combine the information in the second row with the short names in the first row. By using a formula like =CONCATENATE(R1, ” – “,R2) and a little clever copying-and-pasting, the headers can quickly take a more appropriate shape:
Depending on the nature of your file, some further tidying may be necessary, but a little trick like this can get you a long way.
Use headings to ensure your variables get grouped together
There is a range of survey question styles which collect multiple answers from each person. The most common examples include the traditional multiple response question, and single response and multiple response grids. In most cases in your workflow, you will want to have the data from such questions grouped together in Q. The column headers that you use can help Q to identify when a group of variables should be analyzed together. The basic idea is that the column headers should contain an identifiable structure which indicates when variables are meant to go together.
The screenshot in the first section of this article contains one such set of variables for a multiple response question. The column headers are Q1a, Q1b, and Q1c. The common prefix is enough to tell Q that these variables go together.
Consider the case shown below, where we have asked people what aspects they feel are important when deciding what food to get delivered for a night in. The labels only tell us which response option was shown and there is no info in the headers to indicate that the variables are related to one another. These variables would not get grouped together when the data is imported into Q.
A better layout for these variables is as follows. Here, the text from the question has been included in the headers, and this pattern will ensure that Q groups these variables together when the data is imported.
The common prefix in the headers, “How important are the following when ordering delivery food? – “, is enough to allow Q to recognize the structure of this data.
Using Excel files in Q
The tips above will help you get the best file possible Excel file for Q. Even with a tidy file, you may need to complete some other setup once you have imported the file into Q. This includes entering or tidying labels, recoding numeric values, and grouping variables. All of these things are really easy to take care of in Q once you know how!
Importing and checking your data
To figure out what parts of your data need to be tidied, it is good to start by generating a SUMMARY table for all of your survey questions so that you can go through them one-by-one.
- Import your data using File > Data Sets > Add to Project > From File.
- Choose your file and click Open.
- In the Data Import window, you should make sure to select Automatically detect data file structure, and expand the Advanced section and tick Summary Tables. This advanced option will generate a table for everything in your file. You can run this option later on using Automate > Browse Online Library > Preliminary Project Setup > Summary Tables.
The Report on the left side of the Q window will now contain a folder called Summary Tables which contains a table for each question in your files. Now I will go through some common issues and how to address them.
Table shows an Average instead of categories
In this example, the Age question in my Excel file contained numbers for the age groups instead of labels. The table in Q is showing an Average of those numbers instead of showing the percentage of people in each age group.
To show categories instead of an Average you should:
- Right-click on the table.
- Find the name of the question in the context menu (in this case the name is d1).
- Select Restructure Data > Pick One: Mutually Exclusive Categories.
This process, called changing the Question Type, is one of the most fundamental things to learn about controlling your data in Q. For more on this, check out our getting started guide.
Categories don’t have labels
If you have columns with numbers representing categories, like the table above, you will want to add category labels. Labels for categories can easily be added in Q.
To enter labels:
- Right-click on the table and select Values.
- Enter the desired category labels in the Label column.
- Click OK.
Recoding numeric values
When you first import your Excel file, Q will assign numeric values for any categories that it identifies. This is, importantly, different from using SPSS files and other files with metadata, where categories are stored with both a label and a numeric value.
For some categorical data, like Gender, the numeric values are relatively unimportant. However, if your data contains a scale, like satisfaction ratings, then the numeric values can be useful to your analysis in computing an average for the scale among different groups of respondents.
To check and change the numeric values that have been assigned
- Right-click on the table and select Values.
- Change the entries in the Value column as needed.
- Click OK.
In this example, I have a 5-point scale where the categories have been coded as:
- Dislike = 1
- Hate = 2
- Like = 3
- Love = 4
- Neither like nor dislike = 5
This is not a useful set of values for this data. I recode the categories as:
- Hate = -2
- Dislike = -1
- Neither like nor dislike = 0
- Like = 1
- Love = 2
Tidying variable labels
Variable labels come from the column headers in your Excel file. These labels will affect the way items appear in tables, particularly for multiple response questions, and other types of questions that contain multiple variables (like grids). You can access variable label by typing directly into the Label column of the Variables and Questions tab.
If you have a table of labels (for example in another Excel file) you can paste multiple labels at once by right-clicking in the Label column and selecting Paste Labels.
Data appears as Text
When Q sees a column full of text in the Excel file it needs to make a decision about whether the text in the column represents a set of category labels or it represents open-ended responses. Q makes an educated guess based on the number of unique responses in the column, and the lengths of the responses. Sometimes Q can guess incorrectly, particularly if you have a really large brand list.
If Q has not identified your data as being categorical, the table will show all of the responses. To create a new copy of the data with each unique response turned into a category you should:
- Right-click on the table.
- Find the name of the question in the context menu (in this example it is Preferred Cola).
- Select Restructure Data > Pick One: Mutually Exclusive Categories.
This process, called changing the Question Type, is another one of the most fundamental things to learn about formatting your data in Q. For more on this, check out our getting started guide.
Grouping variables
If variables are meant to be grouped, as in a multiple response question or grid, you can group them as follows:
- In the Variables and Questions tab, highlight the rows that you want to group.
- Right-click and choose Set Question.
- Choose an appropriate Name and Question Type, and click OK.
In the following example, I have rating scales for several soft drink brands. They begin as separate Pick One questions, and I group them as a Pick One – Multi question (so that the brand ratings can be shown together). For more on choosing the Question Type, see our getting started guide.
Time to get started
If you follow the steps above to preparing your Excel or CSV file for use in Q you will be ready to get started! These specs should take care of just about everything, and any remaining issues can easily be addressed in Q.